Deep learning is an artificial intelligence (AI) function that mimics the functions of the human brain. It mimics in processing data and creating patterns for use in decision making.
Also, deep learning is a subset of machine learning in artificial intelligence. In addition, it is known as deep neural learning or deep neural network.
It is a machine learning technique which learns features and tasks directly from data. The data can be images, text files or sound.
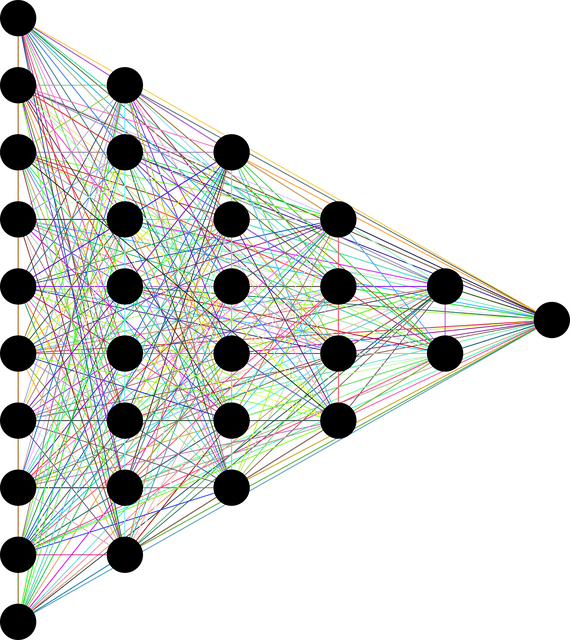
Why Deep Learning Is Important?
Why is deep learning and artificial neural networks so powerful and unique in today’s industry? And above all, why are DL models more powerful than machine learning models? Let me explain it to you.
The first advantage of DL over machine learning is the redundancy of so-called feature information.
The ability to process large numbers of features makes it very powerful. This feature works while dealing with unstructured data.
However, its algorithms can be overkill for less complex issues because large amounts of data need to be accessed to be effective.
Above all, if the data is too simple or incomplete then, it is too easy for DL model to become overfitted and fail to generalize new data.
As a result, here, DL models are not as effective as other techniques.
How Does Deep Learning Work?
Neural networks are the layers of nodes. The network is more in-depth based on the number of its layers.
In an artificial neural network, signals travel between nodes and assign corresponding weights. The final layer compiles the weighted inputs to produce an output.
DL systems require powerful hardware because they have a large amount of data being processed.
It classifies the data with the answers received from a series of binary true or false questions involving highly complex mathematical calculations.
Most importantly, as we understand, DL unravels huge amounts of unstructured data that would normally take humans decades to understand and process.
Advantages
- Features are not required to be extracted ahead of time. This avoids time consuming machine learning techniques.
- Robustness to natural variations in the data is automatically learned.
- Architecture is flexible.
- The same neural network based approach applied to many different applications
- No human intervention needed (automation).
- Wide Applications
Disadvantages
- Time and Resources
- Interpretation of Results
- High error-susceptibility
- It requires very large amount of data in order to perform better than other techniques.
- It is extremely expensive to train due to complex data models.
Summary
In this article, we discovered that deep learning is just very big neural networks on a lot more data, requiring bigger computers.
As a result, we have studied Advantages and Disadvantages of deep learning. Also, this blog helps an individual to understand why one needs to choose deep learning.